Using AI in HR
Key questions for 2024
The use of AI in Talent Management continues to be heavily promoted and claimed to assist in;
- Assessing staff skills and suitability for hiring and promotion
- Creating individualised training programmes to improve productivity
- Career Development
- Analysing employee sentiment for engagement and retention rate improvement
- Automating HR administrative processes
These systems are driving important decisions about people that affect lives and organizational success. It is imperative that we understand what they are, how they work, the very many risks associated with them, and their potential benefits.
Previously we highlighted the perils and positives of the use of AI in talent management – here we take a deeper dive and identify key questions for 2024.
What is AI versus Generative AI?
There are two distinct approaches to artificial intelligence (AI) – the simulation of human intelligence.
The top down approach is the attempt to simulate conscious reasoning, which in humans is mostly language based. The bottom up approach simulates the physical functioning of the brain – its neural connections. These systems are artificial neural networks, that, like humans can learn from experience.
In the top down approach systems are provided with specific rules based models. Robots have a sequence of steps to perform in one or more specific circumstances. Chatbots and intelligent assistants have responses and response sequences to follow for commonly asked questions. IBM’s Watson uses a model of the reasoning steps by a human deciding the next move in a game of chess.
Systems can store and quickly access far more information than humans, so they can handle situations with many variables. This can enable them to be more effective than humans on tasks that do not involve novel circumstances.

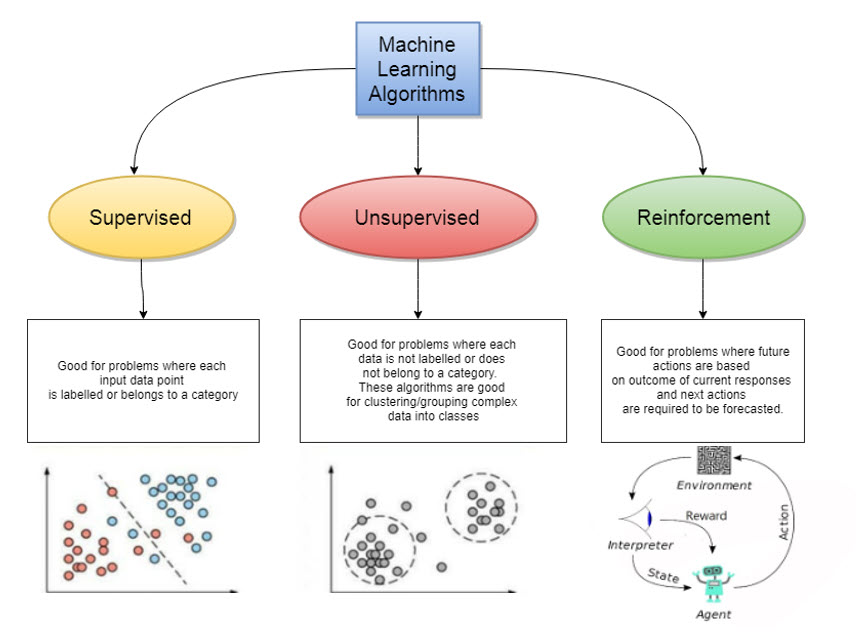
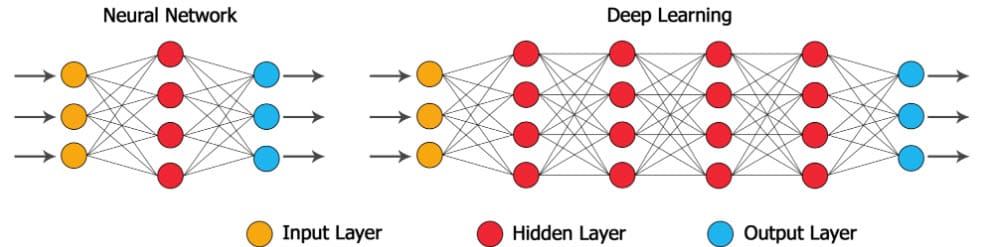
In the bottom up approach systems use learning algorithms, that, like humans, can learn from experience. Typically known as Machine Learning. These systems discern patterns in historical data that they use to predict future outcomes based on currently presented data. For example security risks in physical and on-line situations. In healthcare, machines presented with many labelled images of malignant versus benign tumours (training data), can learn to distinguish the difference in new images presented.
Natural Language Processing models used in HR applications are models of word sequences that are realistic to human use. Probability distributions of word sequences are used to create these models.
There are three types of machine learning;
- Supervised – a human is in the loop to set and clarify rules (categories and labels)
- Reinforcement – learning from mistakes and successes
- Unsupervised – the machine itself discerns the patterns
Artificial neural networks may be used to manage thousands of variables and are of varying depths.
Learning algorithms are based on statistics of ongoing correct categorisations and can never be 100% accurate. Where mistakes are found the algorithm must be corrected and more training data provided.
The launch of ChatGPT saw much enthusiasm on the potential of Generative AI.
This is a very specific use of Machine Learning to create novel output. This output is based on large (enormous) models of patterns and language (LLMs). These are combined with complex algorithms using probability distributions to create new patterns based on the huge amount of data the machine is trained on.
Essentially it combines existing data in new ways.
Despite many red flags such as inaccuracies and over generalised ‘canned’ output, organisations and individuals within them have rapidly adopted these tools to help with language based tasks such as standard letters, emails, promotional communications, blog posts, screen plays, computer code, and even job descriptions.
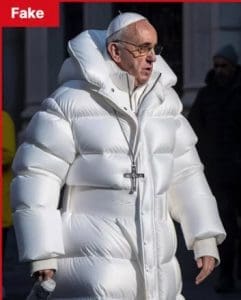
In the creative industries huge datasets of images and other patterns can be used to produce realistic images of people, animals and objects that have never existed, as well as music.
A recent article in Nature describes how Chat GPT4 was used to construct a fictional set of medical research data, not supported by real data, and that was designed to support a scientific hypothesis contrary to most current research. The data set required expert analysis and authentication checks to detect the fabrication.
The difficulty in identifying errors is one of the concerning issues with this technology.
What is Automated Decision Management?
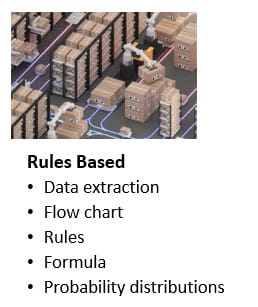
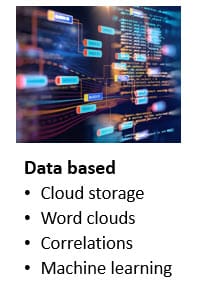
Automated decision making is the delegation of a decision making process to an algorithm. In the past such algorithms were programmed with transparent rules. Weights could be attached to particular data types and points.
There is a spectrum of decision making automation according to how much, if any, participation there is by humans.
At the lower end are decision support systems where decisions are made by humans based on enhanced information and suggestions from a machine – such as transport and service vehicle routing decisions, staff scheduling.
Then there are decisions made mostly by the algorithm but where exceptions from the norm are referred to humans.
Next are the situations where decisions are made by the algorithm but monitored by humans who may adjust the algorithm to optimise decision outcomes. Examples in horticulture systems.
Finally fully automated decision making where the details are decided by the algorithm. An example is Google Adwords fully automated bid system for keywords. The advertising user can input constraints – budget, geography, timings and objectives. There are no published rules for the bidding.
In cases such as this, with the advent of machine learning, the machines themselves find patterns from their training and current data. They use these patterns to evolve their algorithms. Since the algorithms are highly complex and constantly evolving there is no way for users – and also often the creators – to understand the decision making process.
Are AI based decisions effective in Talent Management?
If AI is to be used in Talent Management assessments it must be able to produce sound decisions.
There are two key components
Is the AI Assessment valid?
- The measurement
If the assessment is measuring a specific quality – such as a personality characteristic or cognitive ability – it must be able to demonstrate that this is in fact what is being measured. In comparison with other types of assessment, for example well validated personality tests, the AI decision should produce a similar result. Vendor documentation should provide details.
Most AI assessments don’t measure a single factor but rather a range of predictors. They provide a “criterion measurement”. For example a criterion score that is related to successful job performance or other desirable outcomes such as employee retention. The criterion measurement must be based on properly conducted job analysis for the specific role in question.
- The training data?
The algorithm is trained on an outcome measure – for example job performance. This measure in the training data must be relevant, accurate and unbiased.
This is a major problem for Talent Management decision making. Firstly most organisations lack an objective measure of individual performance. The most commonly used is performance appraisal scores which research shows are more related to the staff/supervisor relationship than to job performance. Secondly, training data should be of individuals similar to the types of applicants it will be used to assess. To achieve that requires small samples – which have low validity when using decision making algorithms based on probability statistics.
Input to AI-based tools comes from a variety of sources which include incumbent workers, job applicants, managers, and organization records of training and performance.
Many vendors offer large training databases that they claim are applicable to all organisations. This is only true if the assessment measures knowledge, skills and abilities that are widely used, and uses the same data across all users. Tools developed in the vendors context may not have predictive value in other settings. Vendors must provide evidence from cross validity studies. Algorithms that are less transparent (e.g., a neural net), or where the algorithm is learning and changing regularly will have more validity issues.

- The collected assessment data
Then the data that is used in the assessment must also be job relevant. AI systems in Talent Management tend to collect data from many sources that are not directly job relevant – such as social media.
When collecting social media data aspects that are relevant to job performance may be missed and data may be included that has no relation to job performance – for example social media likes and images. The volume of this data makes it impractical for subject matter experts to assess its validity.
There are also questions on the validity of facial expressions, gestures and movement in video interviews for job performance.

Is the assessment reliable
Will it produce the same result if re-used. In the case of machine learning there may not be consistency of the applicant and training data used over time .
Validity and reliability problems raise issues of fairness and bias in the use of AI decisions for talent management, and in fact other social decisions such as those in the justice and policing systems.
The model used also has an impact.
The distinction between algorithms intended to estimate an individual’s standing on a particular characteristic and algorithms intended to provide a success score should be considered carefully when determining use of an AI assisted selection system.
The goal of some AI-based applications is to determine a classification to which the data for a specific person fits best (e.g., personality type). In general personality types have little impact on job success. This approach has been questioned because in the absence of a criterion for job success there must be a valid (research based) justification for using the classification in relation to talent management.
Are AI assisted processes fair?

There are many different perspectives of fairness.
Personal perspectives may be based on equality, need (resources allocated based on need) or equity – outcome is proportional to input, those who contribute the most are rewarded with the best outcomes.
People tend to equate fairness with equality – but this is a mistake. People differ on many factors and some are naturally better at certain tasks than others. The aim of Talent Management is to attract, hire and retain those who will perform best, develop over time and remain with the organisation long term.
As such the Talent Management system must successfully identify those with the best skills for the job. Fairness should be taken as a lack of bias in the process.
The OECD includes the transparency of the algorithm as well as its validity and reliability in its definition of fairness of AI decisions.
The very nature of machine learning systems requires large databases of training data. Sampling bias is inevitable because the training data cannot be selected randomly. Some members of the general population are more likely to be selected than others. Sampling bias means this may influence the predictions of the algorithm.
Consideration of fairness must include the assessment process itself. This includes instructions, assessment environment, access to practice material or previews, feedback and opportunity for re-assessment.
Potentially sources of bias in the assessment process include;
- algorithms that are being constantly updated with new data – applicants are not competing on a level playing field – standards are always changing.
- algorithms that include data that is less job relevant – such as social media – use of which is mostly personal.
- inequality of access – for example video interviews disadvantage those with poorer quality equipment and internet connection.
- Some people may be less comfortable and effective in very media rich assessments.
- Facial recognition algorithms have been found to be biased against darker skins.
Ideally the training data and algorithm are continually evaluated to determine the bias ratio – the extent to which its predictions differ from the true outcome.
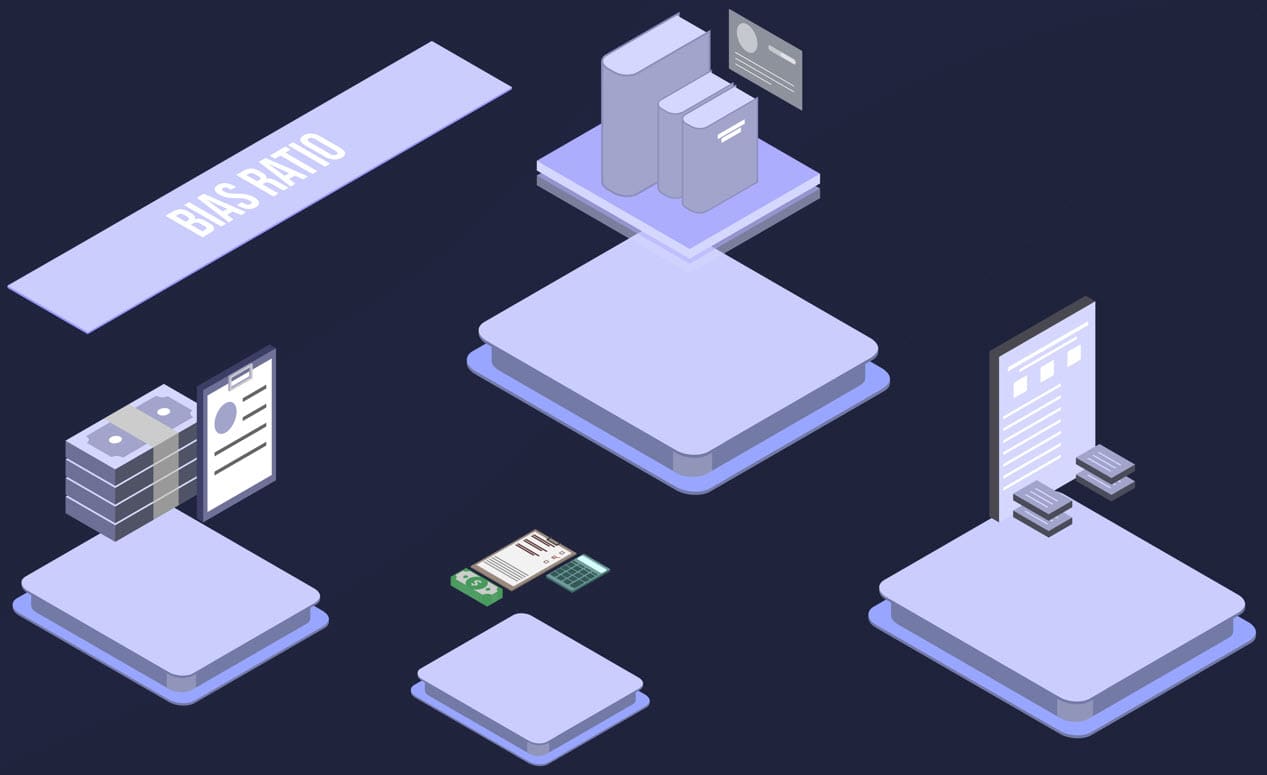
What are the Risks of using AI in HR processes?
Adverse impacts, other legal issues
Automated decision making brings risks of privacy invasion, biased decisions, and discrimination in talent management processes – adverse impact.
The Association for Computing Machinery states that liability rests with organisations using such systems – “even if it is not feasible to explain in detail how the algorithms produce their results.”
The Equal Employment Opportunity Commission recently issued guidance that employers can’t rely on assurances given by makers of AI assisted technology that it complies with civil rights laws.
Requirements on the use of AI in the employment area are rapidly changing with the passage of laws internationally and vary nationally and by state. For example in some jurisdictions privacy regulations may be infringed where individuals are required to provide social media information.
At the least, organisations risk reputational damage if their AI assisted systems make erroneous conclusions
From the individual perspective such problematic decisions may be permanent and often cannot be contested. The possibility of legal recourse for individuals is currently unclear, especially with the lack of transparency in many systems.
Adverse impact is defined as an employment related decision affecting a member of a race, ethnic, religious, age or gender group where success rate is less than 80% of the rate of the most successful group. It can occur-
- Where training data is unbalanced – for example there are many more males than females in IT positions. Unequal representation of groups in the data will cause the algorithm to reflect the most prevalent patterns in the training data — those of majority group members
- Where differences in group mean scores in the training data are not representative of the assessment population.
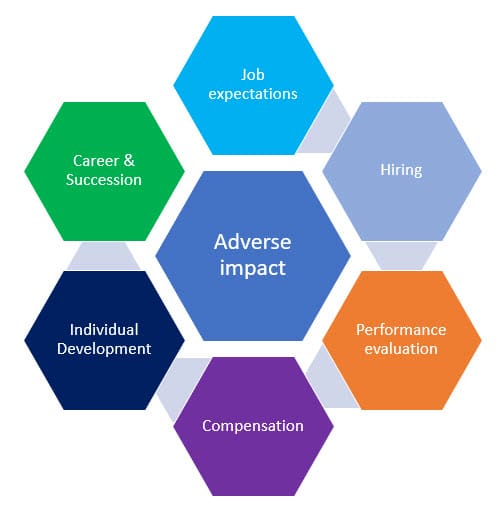

Poor decisions
The characteristics of the data in the system impacts decision quality. Where any of the following issues occur, AI predictions and decision support may be erroneous;
- Differences between individuals in the training data and those in the assessment pool. For example, if organisations are looking to upgrade their skills base, the training data may be of lower skilled incumbents.
- Using data in the algorithm that is not job relevant – social media information, body language, facial expressions and voice tone in interviews. There is as yet no evidence that these factors are related to job performance
- Success criteria in the training data that have low validity and reliability – e.g. supervisor performance ratings
- Unbalanced composition of training data samples biases prediction toward the more common group types.
- Frequent Algorithm changes due to learning, mean that today’s predictions will be different from those in even the recent past. This causes problems for users if not well documented, as well as for validity of retests and other comparisons.
- Assessment format changes can produce score differences and some formats may favour certain subgroups
Mitigating the risks
Many of the risks of using AI in Talent Management can be reduced with the following strategies;
- Use job analysis to understand the work that will be performed and the worker capabilities required to perform this work.
- Ensure that the criteria used in the algorithm are directly relevant to the work (sales, service, error rates, productivity) and psychometrically valid via a criterion-related validation study
- Take into account any task variations from the standard expectation, any geographic differences
- Ensure the training data is balanced – not biased and is representative of the group(s) to be assessed going forward
- Ensure that information that is not relevant to job performance is disregarded
- Ensure that humans are in the loop, that decisions are not completely autonomous. Research shows that humans are capable of identifying cases where machine decisions are wrong.
- Ensure comprehensive admin instructions so that assessments are consistent and all the required information is provided to assessees. Regulatory requirements change frequently and must be monitored closely.
- AI tools must be defensible – this requires transparency – adequate documentation to demonstrate validity. There should be details of data sources – how the information was gathered – both for training and new data.

Attempts to mitigate potential bias can end up violating employment law. Adverse impact programmes that score assessments differently for different groups are an example. Loading extra training data for protected groups may also be problematic.
Finally research shows there are cases where ordinary least squares regression performs better than alternative machine learning models developed on the same data.
What is XAI - Explainable (transparent) AI?


In view of the potential risks it is not surprising that “explainable AI’ is coming to the fore. A range of XAI tools are now available to vendors to make it easier for them to analyse and provide the kind of information required for explainability.
The AI systems marketed for use in Talent Management are complex and therefore to be explainable they should have comprehensive documentation.
There is likely a gap between what IT people and those in Talent Management deem explainable. The documentation must be understandable for non experts and it must enable identification of the reasons for errors in AI system predictions.
At a minimum this documentation should include;
- Details of the training data with summary statistics (means, variability and distribution) of the population groups and all the factors used as inputs to the algorithm
- Details of the algorithms’ scoring process – what factors are considered, how they are weighted, and how often the algorithm is updated, and on what basis it is updated.
- How missing data is handled – for example data such as social media that does not exist for all individuals or interrupted and therefore incomplete interviews
Ideally the documentation also describes –
- how the training data was captured, types and sources
- how it was processed (labelled, categorized)
- its relationship to actual job requirements
- data sufficiency – how the minimum data requirement for training the algorithm was calculated
- how the factors for the algorithm were derived
- the selected machine learning model
- The model’s variables and configurations (hyperparameters) and how they are tuned, configured
- the predictive criteria – a particular characteristic or combined score
- The cross-validation studies used to demonstrate criterion validity including details of the development and validity testing samples.
Vendors may argue that this information is proprietary and that providing it would be allowing competitors to copy or bad actors to game the system. Over time they will need to comply if they want to make sales.
Benefits and Limitations of AI for HR processes
Benefits
It is suggested that the use of AI can reduce transactional HR work, facilitate recruiting, minimise bias, enhance staff retention and talent mobility.
Application of Neuro-Linguistic Programming (NLP) and Review of International Comparative Management Volume 24, Issue 3, July 2023
Computers outperform humans in being able to access huge amounts of data and process it superfast. There is no doubt they can outperform humans on certain types of tasks.
Tasks in which the information is clearly defined – such tasks in Talent Management potentially include;
- Automation of transactional tasks such as onboarding of generic positions.
- Scheduling of staff
- Handling of employment related information requests by chatbots
- Selection of new hires and assessment for promotions and redeployments
- Prediction of staff attrition using sentiment analysis of surveys

Limitations
Generative AI
Where organisations and their staff are using Generative AI – ensure that any resulting product is not violating privacy and intellectual property rights.
When using open platforms like ChatGPT there is a risk that employees could upload proprietary information without realising that it will then be retained and used by the platform as part of its dataset.
Machine Learning
AI systems with Machine Learning are very brittle. They have a very specific context. They must be built by researchers and engineers, with special coding for processing input data, special sets of training data, and a specified learning structure for each new problem domain.
Large Language models are just statistical correlations of language placement in text or speech. There is no underlying model of the world. Human language models are far richer, including real world contexts and the intentions of those communicating.
As a result AI may well identify spurious correlations. For these systems any strong correlation may be taken as cause and effect. Lacking the rich world models that humans have, AI algorithms do not recognise implausibility.
For AI assisted decision making the quality of the data is paramount. Garbage in means Garbage Out (GIGO)
As we have seen much of the data that is used in these systems is biased, it may have missing data points, too little training data, and it may lack important variables.
Whereas humans with their extensive world models and ability to learn across many domains can infer performance on a set of tasks from observing performance on one of them. AI systems cannot do this. Nor can they answer questions that have never been asked before.
As a result, algorithms fail in many situations where humans can reliably come to the right decision. If we use AI in Talent Management we must understand that mistakes will happen and that some of these mistakes will be unexpected from a human perspective. They could be missed due to complete automation and or a lack of transparency and be used to make erroneous decisions.
Therefore it is critical to carefully consider the best uses of AI in Talent Management and to select the appropriate level of automation. For some transactional types of tasks full automation is feasible. For others partial automation as decision support is advisable with the algorithm providing advice and the human making the decision. For situations that involve social and linguistic complexity AI technology is not yet sufficiently advanced to be worth the risks.
How do staff perceive AI in HR processes?
Recent research shows that in staff selection processes, automated (versus in person) assessments can cause negative perceptions of fairness and validity. Some find the process emotionally challenging and automated feedback is not well accepted. This has a flow on effect to make the organization less attractive, and negatively affects job acceptance intentions.
In HR mgmt. The International Journal of Human Resource Management 2022, VOL. 33, NO. 6, 1065–1097
Artificial intelligence – challenges and opportunities for international HRM: a review and research agenda.
Pawan Budhwara, et al.
Research into the use of chatbots for automating many routine HR tasks and information requests has shown mixed results.
The HR staff themselves have some reservations – there are difficulties in implementing the technology and in keeping the information it provides up to date. This can be time consuming. Naturally also it may be seen as a threat to some HR jobs. They felt that ideally the chatbot answers routine questions while HR staff provide guidance at the individual level.
On the positive side use of a chatbot does free up HR time for more valuable activities and enables over time the preparation of better answers to FAQS.
From the employee perspective the key information sought relates to organizational policy, codes of conduct and other conditions of employment. Questions regarding leave, work hours, compensation and benefits , as well as operational questions about access cards, parking spaces, canteen, meeting rooms, computers, and where to find information of various types. Employee reactions were mixed – and related to their expectations of the chatbot.
Those with change fatigue were less likely to find the chatbot useful – as were those that asked very specific questions or personal questions such as balance of leave remaining.

For decision management an MIT study found that there is no common response to decision support AI. They found surprisingly, given the identical AI provided information, that individuals make quite different choices. For example some executives invested 18% more than others on initiatives, based on the identical AI advice.
These decisions can have a significant financial impact on the organisation.